Why i have many negprobe-wtx rows in the count files
Introduction
Nanostring GeoMx Digital Spatial Profiling (DSP) gives a sophisticated approach for integrating both fashionable pathology and molecular profiling to generate unique and reliable spatial multiomics records. This information, which why i have many negprobe-wtx rows in the count files can be derived from complete tissue sections, formalin-constant paraffin-embedded (FFPE), or clean frozen samples, captures each RNA and protein expression. These tissue samples are subjected to staining and imaging, followed via the quantification of decided on areas or regions of interest, either the use of the nCounter Analysis System or an Illumina Sequencer.
GeoMx RNA assays are designed to offer each quantitative and spatial insights into gene expression from a single tissue segment. These assays cowl a vast variety of transcriptomes, taking into account the size of as much as the entire transcriptome from both FFPE or fixed clean frozen tissues. Commonly used gene panels, which include the Cancer Transcriptome Atlas (CTA) with approximately 1800 genes and the Whole Transcriptome Atlas (WTA) with about 18,000 genes, provide complete data for cancer studies and other molecular studies.
The information generated by way of the DSP pipeline undergoes a thorough preprocessing section the use of the GeoMx Data Analysis Suite (DSPDA). This suite guarantees best manage by way of doing away with low-acting probes and calculating a quality metric called the Limit of Quantification (LOQ) based totally on negative probes. To hold records consistency and accuracy, normalization is generally completed in line with guidelines encouraged via Nanostring. However, it is essential to observe why i have many negprobe-wtx rows in the count files that Q3-normalized records isn’t perfect for bioinformatics workflows. Instead, it is encouraged to utilize the probe-corrected counts (probeQC), which adjust for any systematic mistakes which can stand up at some stage in the DSP run, ensuring the most reliable and correct effects.
This workflow gives the very best wellknown of spatially resolved records, allowing researchers to benefit an in-intensity knowledge of tissue architecture and molecular expression with regards to numerous organic and disease states.
Nanostring Library Preparation and Exploratory Analysis
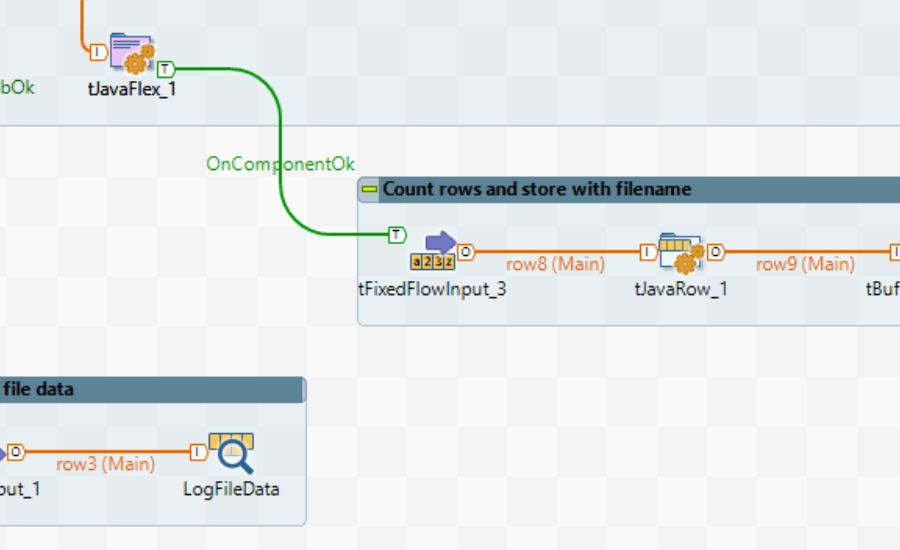
In the method of studying Nanostring facts, uncooked probe counts and area of hobby (ROI) annotation tables had been derived from GeoMx-DSP. Upon examining the records, we observed no proof of biases in library sizes or nucleus counts throughout the different conditions or annotations (Supplementary Fig. 1a). Using a scatter plot to assess sequencing saturation in opposition to alignment, we excluded seven ROIs that did not meet the ninety% threshold for both metric (Supplementary Fig. 1b, Supplementary Table 2).
For exploratory information analysis, we utilized the standR package deal and applied three normalization methods: Counts according to Million (CPM), Q3 normalization, and Remove Unwanted Variation (RUV4) (Supplementary Fig. 1c–e). All 3 methods produced comparable distributions of relative log expression throughout the ROIs, as shown in the left panels of the figures. However, most effective the RUV4 method effectively removed the patient batch impact, which was obtrusive in the important aspect analysis (PCA) plots. While the PCA plots based totally on CPM or Q3 normalization in general showed clustering by way of patients, RUV4 normalization enormously grouped the ROIs according to disease situations—everyday manage, idiopathic pulmonary fibrosis (IPF), and non-particular interstitial pneumonia (NSIP) (Supplementary Fig. 1e, proper). This is similarly why i have many negprobe-wtx rows in the count files emphasised through the elimination of batch outcomes from the time of sample instruction, as seen in Supplementary Fig. 1f.
To cope with capability confounding factors at some point of differential gene expression evaluation, we hired the limma-voom pipeline for downstream analysis. The weight matrices derived from the RUV4 normalization had been covered as covariates inside the linear version to account for any batch effects (Methods segment).
Gene Expression Patterns in LMEs Across Different Conditions
With this dataset, we had been able to discover tissue heterogeneity that won’t be observable via bulk RNA sequencing methods. We focused on specific disease situations and their corresponding LMEs (local microenvironments) to pick out genes and gene sets with good sized variations between annotations. In IPF, we discovered three awesome LME clusters: uninvolved areas, fibroblastic foci (f.Foci), and fibrotic/lymphoid regions (Fig. 2a).
To uncover the maximum considerable molecular distinctions among these LMEs, we accomplished pairwise comparisons and highlighted genes with the most awesome absolute log2 fold modifications and altered P-values. Volcano plots displayed these variations, with the −log adjusted P-fee plotted in opposition to the log2 fold exchange for every contrast (Fig. 2b). Collagen-related genes, which includes COL1A1, COL1A2, COL5A1, COL8A1, and COL3A1, were located to be notably upregulated in fibroblastic foci compared to both fibrotic and uninvolved regions. In assessment, surfactant genes like SFTPA2, SFTPB, and SFTPC exhibited decreased expression in each fibrotic and fibroblastic areas relative to uninvolved regions (Supplementary Table S3).
This evaluation highlights the electricity of spatial why i have many negprobe-wtx rows in the count files multiomics in understanding tissue-precise gene expression styles and the molecular variations associated with disease progression.
Comparison between LMEs across disorder conditions
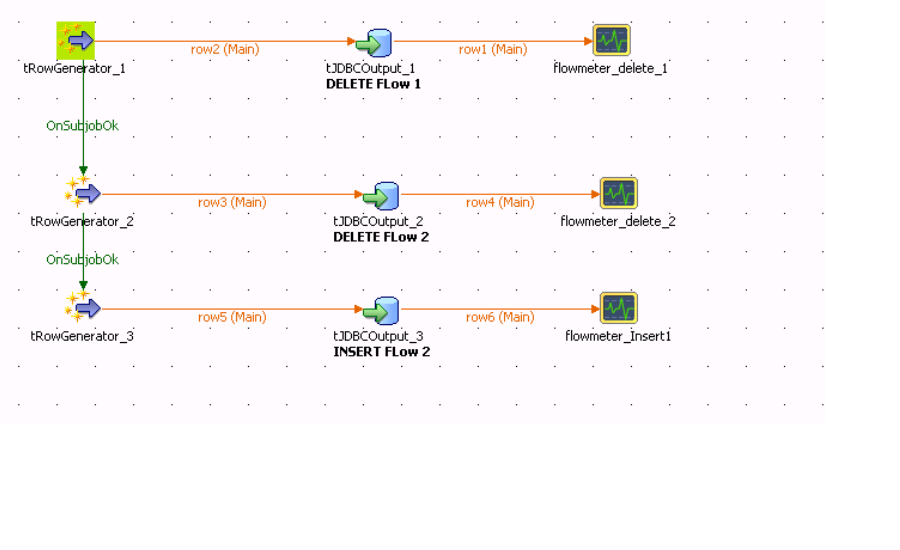
In this analysis, we aimed to examine fibrotic areas throughout distinct sickness conditions to find their awesome gene expression styles, notwithstanding sharing comparable pathological features. The vital and peripheral fibrotic regions of NSIP and the fibroblastic foci (f.Foci) of IPF fashioned wonderful clusters, even as fibrotic areas in both IPF and CHP largely overlapped (Fig. 3a). To identify the most tremendous variations between those regions, we centered at the top 50 differentially expressed genes, which have been ranked by their F-information.
The variability in gene expression between character regions of hobby (ROIs) was assessed and visualized the use of a heatmap, with Z scores indicating normalized expression levels (Fig. 3b, Supplementary Table S9). To similarly categorize the genes, an agglomerative clustering technique was employed, ensuing in clusters. The first cluster, proven in orange, consisted of genes that had been downregulated in each IPF (in its fibrotic and f.Foci areas) and CHP (fibrotic regions) whilst as compared to the primary/peripheral fibrotic regions of NSIP. The 2nd cluster, highlighted in turquoise, contained genes why i have many negprobe-wtx rows in the count files together with COL6A3, COL1A1, and COL8A1, which have been upregulated specially in the f.Foci areas of IPF.
To benefit deeper insights, spatial deconvolution was applied, revealing that a number of the differentially expressed genes overlapped with differences in cell composition (Supplementary Fig. 3a,b). However, genes which include MXRA5, ASPN, COL12A1, and CD248 were discovered to be upregulated in IPF f.Foci, independent of cellular composition (Supplementary Table S10).
Further evaluation using over-illustration checks of pairwise comparisons highlighted that both IPF and CHP notably downregulated gene sets related to antigen processing and presentation, immune cell characteristic, and surfactant homeostasis whilst as compared to the critical/peripheral fibrotic regions of NSIP (Fig. 3c). Many of those genes appeared inside the orange cluster of the heatmap (Supplementary Fig. 3e). Interestingly, gene sets associated with extracellular matrix organisation have been notably upregulated within the f.Foci regions of IPF while in comparison to each CHP and NSIP fibrotic areas, which is steady with the upregulation of collagen genes found in the turquoise cluster of the heatmap (Fig. 3b).
These findings offer treasured insights into the particular gene expression profiles that signify fibrotic and fibroproliferative areas in IPF, CHP, and NSIP, emphasizing the special molecular functions of those sickness conditions.
Facts:
1. Nanostring GeoMx Digital Spatial Profiling (DSP):
- Purpose: Integrates pathology and molecular profiling for spatial multiomics, capturing both RNA and protein expression from why i have many negprobe-wtx rows in the count files tissue samples (FFPE, frozen).
- Applications: Common gene panels such as the Cancer Transcriptome Atlas (CTA) with ~1800 genes and the Whole Transcriptome Atlas (WTA) with ~18,000 genes are widely used.
- Preprocessing: Data undergoes preprocessing through the GeoMx Data Analysis Suite (DSPDA) to remove low-performing probes and calculate the Limit of Quantification (LOQ).
- Normalization: Probe-corrected counts (probeQC) are recommended for accurate results, as Q3-normalized data isn’t ideal for bioinformatics workflows.
2. Nanostring Library Preparation and Exploratory Analysis:
- Data Sources: Raw probe counts and Region of Interest (ROI) annotation tables derived from GeoMx-DSP.
- Quality Control: Seven ROIs were excluded based on sequencing saturation and alignment metrics.
- Normalization Methods: Counts per Million (CPM), Q3 normalization, and Remove Unwanted Variation (RUV4) were applied. RUV4 successfully removed batch effects, and normalization with RUV4 grouped why i have many negprobe-wtx rows in the count files ROIs by disease condition (IPF, NSIP, and controls).
3. Gene Expression Patterns in LMEs Across Different Conditions:
- Tissue Heterogeneity: The study revealed tissue-specific molecular variations not detectable by bulk RNA sequencing.
- IPF LMEs: Three major local microenvironments (LMEs) were identified: uninvolved areas, fibroblastic foci (f.foci), and fibrotic/lymphoid regions.
- Gene Expression: Collagen-related genes (COL1A1, COL1A2, COL5A1, COL8A1, COL3A1) were upregulated in fibroblastic foci, while surfactant genes (SFTPA2, SFTPB, SFTPC) were downregulated in fibrotic and fibroblastic regions.
4. Comparison Between LMEs Across Disease Conditions:
- Cluster Analysis: Fibrotic regions in NSIP and f.foci in IPF formed distinct clusters, while fibrotic areas in IPF and CHP largely overlapped.
- Differential Gene Expression:
- Top 50 differentially expressed genes were selected, with clusters showing different gene expression profiles between IPF, CHP, and NSIP.
- Downregulated genes: IPF (fibrotic and f.foci regions) and CHP showed downregulation of genes related to immune response, antigen processing/presentation, and surfactant homeostasis.
- Upregulated genes: In f.foci of why i have many negprobe-wtx rows in the count files IPF, collagen-related genes (COL6A3, COL1A1, COL8A1) were upregulated, suggesting increased extracellular matrix (ECM) organization.
- Spatial Deconvolution: The differential expression of certain genes was independent of cell composition (e.g., MXRA5, ASPN, COL12A1, CD248 in IPF f.foci).
5. Insights and Conclusion:
- The study provides valuable insights into the molecular profiles of fibrotic and fibroproliferative regions in IPF, CHP, and NSIP.
- It highlights the importance of spatially resolved data for understanding disease-specific molecular functions, offering a better understanding of tissue architecture and disease progression.
These facts underscore the technical sophistication of Nanostring GeoMx DSP in providing spatially resolved, multi-omic insights into tissue samples, especially in understanding the gene expression differences between disease conditions like IPF, NSIP, and CHP.
Summary:
This article discusses the use of Nanostring GeoMx Digital Spatial Profiling (DSP) to analyze tissue samples and uncover spatial multiomics why i have many negprobe-wtx rows in the count files data, providing insights into gene and protein expression in tissue architecture and disease states. The GeoMx RNA assays offer a comprehensive approach to studying gene expression, especially in the context of cancer and other molecular diseases. The data is preprocessed using the GeoMx Data Analysis Suite to ensure high-quality results, with probe-corrected counts recommended for better accuracy.
Exploratory analysis using the standR package and various normalization methods showed that RUV4 normalization effectively removed batch effects and allowed better classification of samples by disease condition. Differential gene why i have many negprobe-wtx rows in the count files expression analysis using the limma-voom pipeline helped uncover tissue heterogeneity, revealing molecular distinctions between different local microenvironments (LMEs) in diseases like idiopathic pulmonary fibrosis (IPF).
The study identified specific gene expression patterns in IPF, particularly in fibroblastic foci and fibrotic/lymphoid regions, where collagen-related genes were highly upregulated. A comparison between LMEs across different diseases, including IPF and non-specific interstitial pneumonia (NSIP), revealed distinct gene expression patterns in fibrotic areas, with notable differences in gene sets related to immune response, antigen presentation, and extracellular matrix organization. These findings highlight the value of spatial multiomics in understanding disease progression and tissue-specific gene expression.
FAQs:
1. What is Nanostring GeoMx Digital Spatial Profiling (DSP)?
Nanostring GeoMx DSP is a high-throughput why i have many negprobe-wtx rows in the count files platform that allows researchers to analyze gene and protein expression across different regions of tissue samples, providing detailed spatially resolved data. This platform is capable of profiling RNA and protein expression from formalin-fixed paraffin-embedded (FFPE) or fresh frozen tissue samples, making it an important tool for spatial multiomics research.
2. How does the Nanostring GeoMx DSP work?
GeoMx DSP works by first staining tissue sections and imaging them to capture the molecular profile. Researchers then quantify the RNA and protein expression from selected areas or regions of interest (ROIs) using either the nCounter Analysis System or an Illumina Sequencer. This process generates spatially resolved data that reflects the tissue architecture and molecular composition of the sample.
3. What are the main benefits of using GeoMx DSP in research?
The primary benefits of GeoMx DSP include its ability to integrate both pathology and molecular profiling, allowing for comprehensive analysis of gene expression in spatial context. This enables researchers to explore tissue heterogeneity that may be missed in bulk RNA sequencing, making it invaluable for cancer research, fibrosis studies, and other diseases with complex tissue landscapes.
4. What types of samples can be used in GeoMx DSP?
GeoMx DSP can process a wide variety of tissue samples, including formalin-fixed paraffin-embedded (FFPE) tissues and fresh frozen tissues. It is versatile and can be used on both small and large tissue sections, which why i have many negprobe-wtx rows in the count files provides flexibility for different experimental needs.
5. What is the role of the GeoMx Data Analysis Suite (DSPDA)?
The GeoMx DSPDA is a software tool used for processing and analyzing the data generated by GeoMx DSP. It includes features like quality control (QC) to remove low-performing probes and normalize the data, ensuring that the results are accurate and reliable. The DSPDA calculates the Limit of Quantification (LOQ) to assess probe performance and ensures that the data is suitable for downstream analysis.
6. How does RUV4 normalization help in data analysis?
RUV4 (Remove Unwanted Variation) normalization helps to eliminate batch effects, which can arise from differences in sample preparation or sequencing. This normalization method ensures that the data is not biased by technical artifacts why i have many negprobe-wtx rows in the count files, leading to more accurate results in downstream analyses, such as principal component analysis (PCA).
7. What is the importance of spatial multiomics in disease research?
Spatial multiomics allows researchers to examine the gene expression profiles of different regions within a tissue, providing insights into tissue-specific molecular mechanisms and disease progression. This is especially useful in complex diseases like cancer and fibrosis, where the tissue architecture plays a significant role in disease pathogenesis.
8. How does the study of LMEs (local microenvironments) aid in understanding disease progression?
By focusing on LMEs, researchers can identify distinct molecular signatures in different tissue areas, such as fibrotic foci in idiopathic pulmonary fibrosis (IPF). These insights reveal how specific regions of tissue contribute to disease progression, helping to identify potential therapeutic targets or biomarkers.
9. What is the significance of collagen-related genes in IPF research?
Collagen-related genes, such as COL1A1, COL1A2, and COL3A1, play a key role in the development of fibrosis. In IPF, these genes are upregulated in fibroblastic foci, which are areas of tissue where fibrosis is actively progressing. Understanding the expression patterns of these genes provides important information about the why i have many negprobe-wtx rows in the count files molecular mechanisms driving fibrosis and potential therapeutic interventions.
10. What findings were uncovered in the comparison between LMEs across disease conditions?
The comparison between different fibrotic regions in diseases like IPF and non-specific interstitial pneumonia (NSIP) revealed that while these conditions share some pathological features, they also have distinct molecular profiles. For example, certain genes were found to be upregulated in specific areas like the fibroblastic foci of IPF, which could offer insights into the unique disease mechanisms in these conditions.
11. How does spatial deconvolution contribute to the analysis?
Spatial deconvolution helps to separate the molecular signatures of different cell types within the tissue, allowing for a deeper understanding of how gene expression varies across cell populations. This approach reveals whether the differential gene expression observed in disease areas is due to changes in cellular composition or intrinsic changes in the tissue itself.
12. What is the significance of gene sets related to extracellular matrix organization in IPF?
In IPF, gene sets related to extracellular matrix (ECM) organization, such as collagen genes, are significantly upregulated in fibroblastic foci. This why i have many negprobe-wtx rows in the count files suggests that the ECM plays a crucial role in the progression of fibrosis and highlights potential targets for therapies aimed at modulating ECM production or remodeling in IPF.
13. What future directions do you foresee for spatial multiomics technologies like GeoMx DSP?
As spatial multiomics technologies continue to evolve, they will likely offer even more precise insights into tissue heterogeneity and disease progression. Future advancements may include better integration of RNA and protein data, higher resolution imaging techniques, and the ability to analyze more complex disease states, paving the way for more personalized and effective therapeutic strategies.
Read More Information About Information At royalbucks.net